Table Of Content
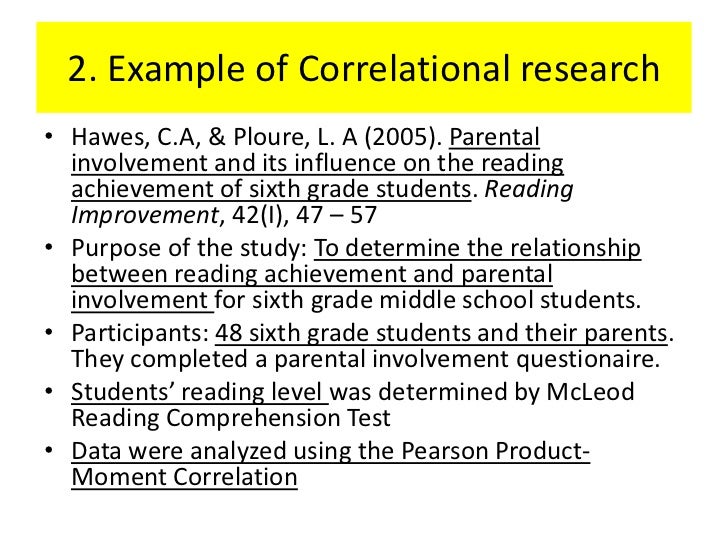
For example, a researcher might evaluate the validity of a brief extraversion test by administering it to a large group of participants along with a longer extraversion test that has already been shown to be valid. This researcher might then check to see whether participants’ scores on the brief test are strongly correlated with their scores on the longer one. Neither test score is thought to cause the other, so there is no independent variable to manipulate.
Eight students advance to 3MT finals
A confounding variable is a third variable that influences other variables to make them seem causally related even though they are not. Instead, there are separate causal links between the confounder and each variable. Instead of collecting original data, you can also use data that has already been collected for a different purpose, such as official records, polls, or previous studies. You have developed a new instrument for measuring your variable, and you need to test its reliability or validity. Free and low-cost resources are available to researchers at all levels through academic institutions, museums, and data repositories around the world. Many areas of psychological research benefit from analyzing studies that were conducted long ago by other researchers, as well as reviewing historical records and case studies.
Validity
Only participants who were able to identify a target meeting these criteria were allowed to proceed to the study, where they were asked to provide the initials for the person so that the remaining questions could be personalized. Vandenbroucke et al. (2014) published an expanded version of the Strengthening the Reporting of Observational Studies in Epidemiology (strobe) statement to improve the reporting of observational studies that can be applied in eHealth evaluation. It is made up of 22 items, of which 18 are common to cohort, case-control and cross-sectional studies, with four being specific to each of the three designs. The 22 reporting items are listed below (for details refer to the cited reference).
Cross-Sectional Correlational Study
While reaching out to old friends may be practical, this strategy may not be enacted because various psychological hurdles hinder people’s willingness to connect with others25. Indeed, recent work indicates that people overestimate the awkwardness of reaching out to an old friend and underestimate the appreciation and positive feelings such acts foster26,27,28. In addition, people misestimate the outcomes of other social acts involving other social partners.
4 Reasons why Correlation does NOT imply Causation by Ines Lee - Towards Data Science
4 Reasons why Correlation does NOT imply Causation by Ines Lee.
Posted: Sun, 18 Apr 2021 07:00:00 GMT [source]
To explore causal relationships between variables
Finally, in Study 7, we provide evidence for an intervention that effectively increases reaching out to old friends - a behaviour that has informational and well-being benefits. A growing body of research demonstrates that people are unwilling to talk to strangers and avoid opportunities to do so. Indeed, despite several studies demonstrating that brief conversations with strangers can promote one’s happiness and belonging29,30, people report both avoiding and dreading these conversations due to a number of fears.
1 Data Collection in Correlational Research
Correlational studies are similar to comparative studies in that they take on an objectivist view where the variables can be defined, measured and analyzed for the presence of hypothesized relations. As such, correlational studies face the same challenges as comparative studies in terms of their internal and external validity. Of particular importance are the issues of design choices, selection bias, confounders, and reporting consistency. Again, because neither independent variable in this example was manipulated, it is a correlational study rather than an experiment.
The best they can do is show patterns of relationships that are consistent with some causal interpretations and inconsistent with others. Naturalistic observation is an approach to data collection that involves observing people’s behaviour in the environment in which it typically occurs. Ethically, this is considered to be acceptable if the participants remain anonymous and the behaviour occurs in a public setting where people would not normally have an expectation of privacy. Naturalistic observation is an approach to data collection that involves observing people’s behavior in the environment in which it typically occurs. Ethically, this is considered to be acceptable if the participants remain anonymous and the behavior occurs in a public setting where people would not normally have an expectation of privacy.
An example is a study by Brett Pelham and his colleagues on “implicit egotism”—the tendency for people to prefer people, places, and things that are similar to themselves (Pelham, Carvallo, & Jones, 2005). In one study, they examined Social Security records to show that women with the names Virginia, Georgia, Louise, and Florence were especially likely to have moved to the states of Virginia, Georgia, Louisiana, and Florida, respectively. Researchers are expected to demonstrate the interrater reliability of their coding procedure by having multiple raters code the same behaviors independently and then showing that the different observers are in close agreement. Correlational research is useful for identifying patterns and making predictions but cannot establish causation. Instead, it helps researchers to better understand the nature of the relationship between variables and to generate hypotheses for further investigation.
Some socially poor but also some socially rich adolescents feel closer to their friends after using social media
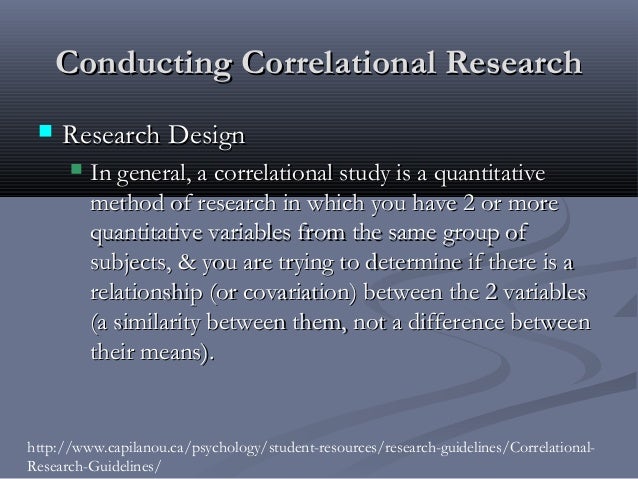
Naturalistic observation is a type of field research where you gather data about a behaviour or phenomenon in its natural environment. Surveys are a quick, flexible way to collect standardised data from many participants, but it’s important to ensure that your questions are worded in an unbiased way and capture relevant insights. Correlational research can be used to assess whether a tool consistently or accurately captures the concept it aims to measure. That helps you generalise your findings to real-life situations in an externally valid way. There are several actions that could trigger this block including submitting a certain word or phrase, a SQL command or malformed data. By Kendra Cherry, MSEdKendra Cherry, MS, is a psychosocial rehabilitation specialist, psychology educator, and author of the "Everything Psychology Book."
Regression analysis can help identify the strength and direction of the relationship between variables, as well as the degree to which one variable can be used to predict the other. Observational studies involve observing and recording the behavior of participants in natural settings. Researchers can use observational studies to examine the relationships between variables such as social interactions, group dynamics, and communication patterns. A negative correlation occurs when one variable increases while the other decreases. For example, there is a negative correlation between the number of hours spent watching TV and physical activity level. The more time a person spends watching TV, the less physically active they are likely to be.
Factor analysis can help identify underlying factors that influence the relationship between two variables. Archival data involves using existing data sources such as historical records, census data, or medical records to explore the relationships between variables. Archival data is useful for investigating the relationships between variables that cannot be manipulated or controlled.
As a final example, researchers Peter Rentfrow and Samuel Gosling asked more than 1,700 college students to rate how much they liked 14 different popular genres of music (Rentfrow & Gosling, 2008). They then submitted these 14 variables to a factor analysis, which identified four distinct factors. Although the strong positive relationship they found between these two variables is consistent with their idea that hassles cause symptoms, it is also consistent with the idea that symptoms cause hassles or that some third variable (e.g., neuroticism) causes both. When researchers study relationships among a large number of conceptually similar variables, they often use a complex statistical technique called factor analysis. As a final example, researchers Peter Rentfrow and Samuel Gosling asked more than 1,700 university students to rate how much they liked 14 different popular genres of music (Rentfrow & Gosling, 2008)[4]. For example, Allen Kanner and his colleagues thought that the number of “daily hassles” (e.g., rude salespeople, heavy traffic) that people experience affects the number of physical and psychological symptoms they have (Kanner, Coyne, Schaefer, & Lazarus, 1981).
Researchers use path analysis to test causal models and identify direct and indirect effects between variables. Another approach to correlational research is the use of archival data, which are data that have already been collected for some other purpose. They found that people in some countries walked reliably faster than people in other countries. For example, people in Canada and Sweden covered 60 feet in just under 13 seconds on average, while people in Brazil and Romania took close to 17 seconds. The quantities b1, b2, and so on are regression weights that indicate how large a contribution an independent variable makes, on average, to the dependent variable. Specifically, they indicate how much the dependent variable changes for each one-unit change in the independent variable.
For example, instead of simply measuring how much people exercise, a researcher could bring people into a laboratory and randomly assign half of them to run on a treadmill for 15 minutes and the rest to sit on a couch for 15 minutes. Although this seems like a minor change to the research design, it is extremely important. Now if the exercisers end up in more positive moods than those who did not exercise, it cannot be because their moods affected how much they exercised (because it was the researcher who used random assignment to determine how much they exercised). Likewise, it cannot be because some third variable (e.g., physical health) affected both how much they exercised and what mood they were in. Thus experiments eliminate the directionality and third-variable problems and allow researchers to draw firm conclusions about causal relationships.
They have limited generalizability to the population at large in routine settings (Fleurence, Naci, & Jansen, 2010). As such, correlational studies, especially those involving the use of routinely collected ehr data from the general population, have become viable alternatives to rcts. There are advantages and disadvantages to each of the three design options presented above. While correlational research does not involve manipulating variables, researchers can use experimental design to establish cause-and-effect relationships between variables. Experimental design involves manipulating one variable while holding other variables constant to determine the effect on the dependent variable. Naturalistic observation is an approach to data collection that involves observing people’s behaviour in the environment in which it typically occurs.
No comments:
Post a Comment